Back to Blog
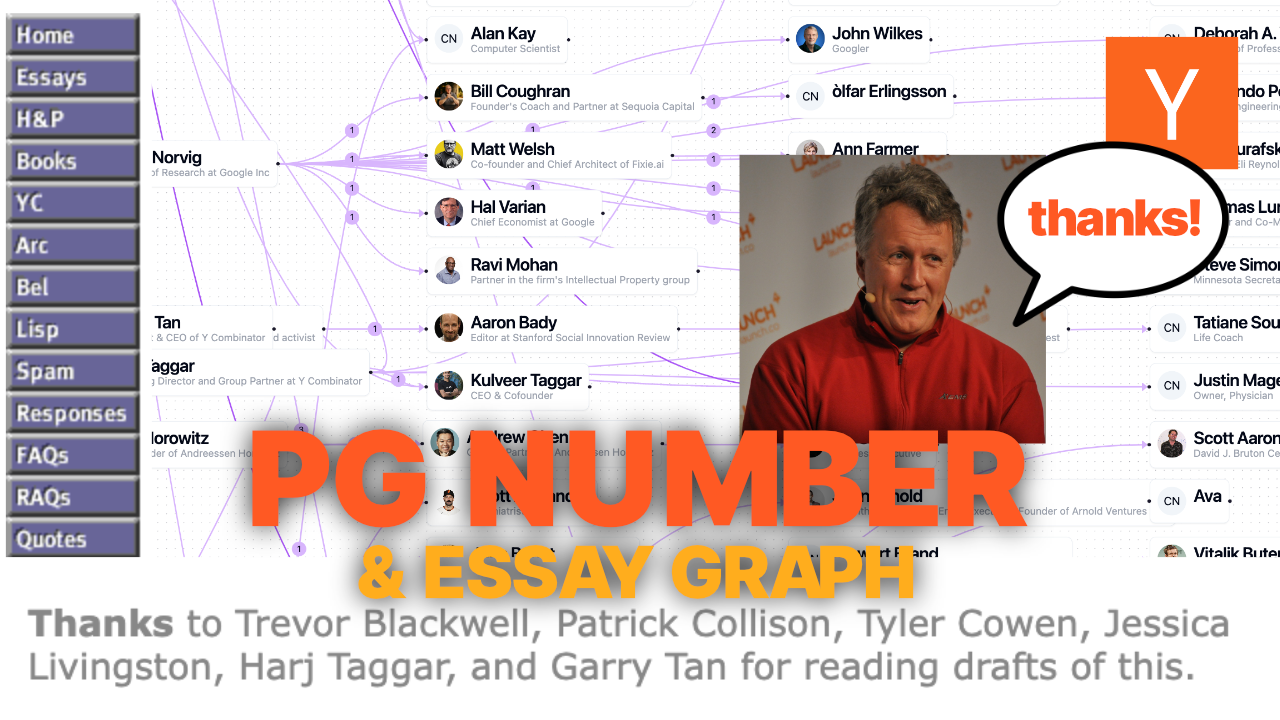
What's your PG Number?
How I built a recursive graph of everyone Paul Graham has thanked in his essays
5 Dec 2023 • 4 min read • 3 mins coding time
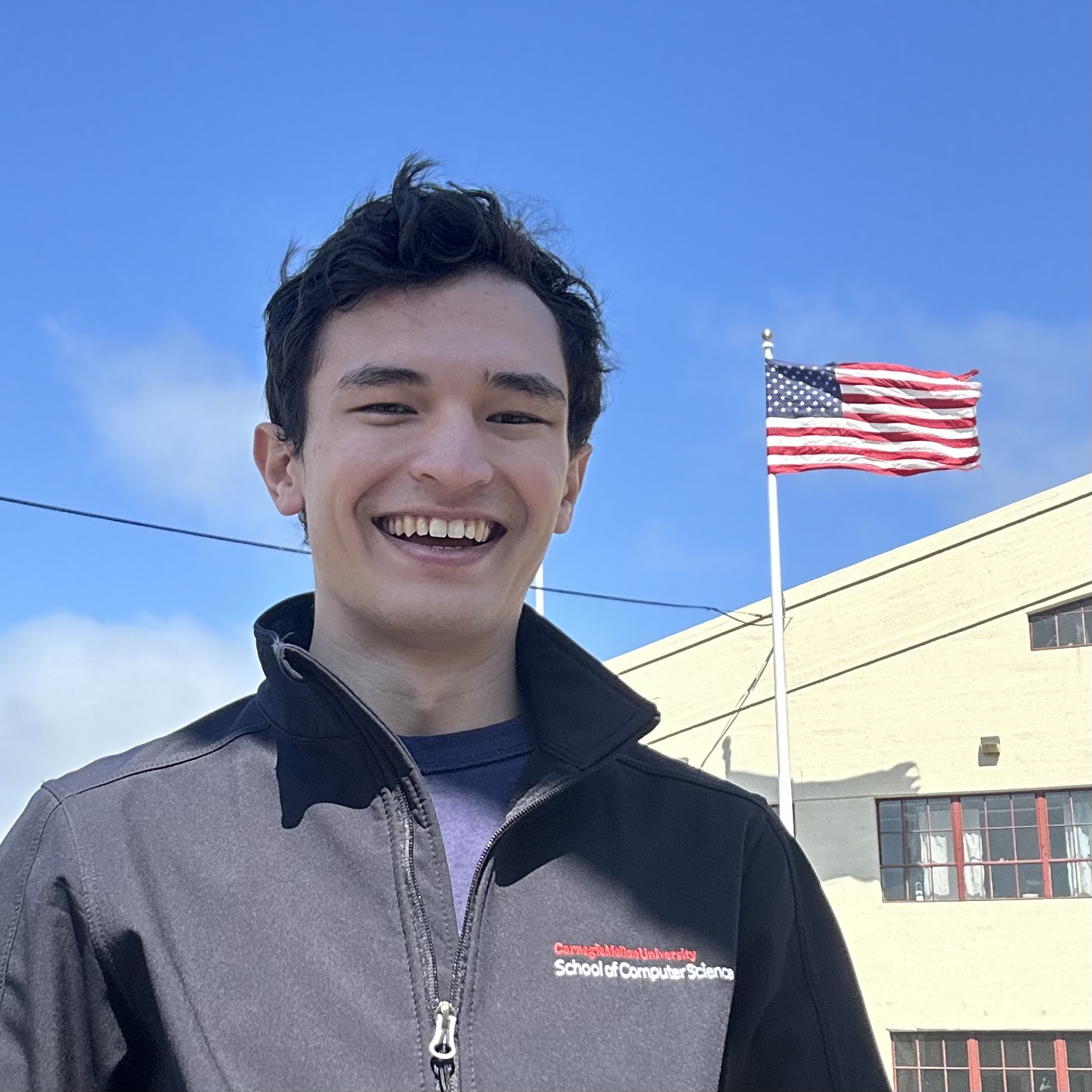
Mike Gee
Context
This is how I built PG Number, the "collaborative distance" from Paul Graham.
Paul Graham was a co-founder of Y Combinator. He is known for his essays on startups and programming.
At the end of each of his essays, he thanks people who have read drafts of the essay and given feedback. I was curious to see these in aggregate.
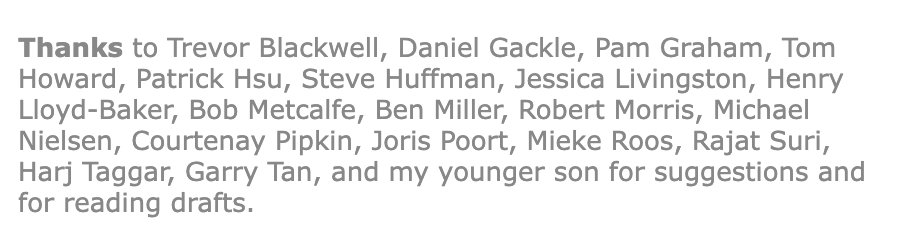
I decided to do the following:
- Scraped all the essays from his website
- Extracted all of the thanked people using LLMs
- Searched for all of the thanked people's blogs & filtered using LLMs
- Recursively extracted all of the thanked people from their blogs
Sourcing the Data
Donloading the Website & Crawling the Data
I started by crawling Paul Graham's Website. I wanted to quickly visit every page and download the HTML.
import webtranspose as webt crawl = webt.Crawl( 'http://paulgraham.com/', max_pages=1000, ) crawl.queue_crawl() print(crawl.crawl_id)
Classifying Web Pages & Extracting Thanked People
I then looped through all of the pages and did the following:
- Classify whether the page is a blog or not
- Extract the people PG thanks
I did this using this schema:
schema = { 'page classification': ['blog / essay', 'other type of page'], 'people thanked': { 'type': 'array', 'items': { 'person name': 'string', 'reason mentioned': ['thanked for reading drafts of this blog / essay', 'other kind of praise', 'other reason'] } } }
I then passed this schema into Web Transpose.
import webtranspose as webt scraper = webt.Scraper(schema) essays = [] for url in crawl.get_visited(): page = crawl.get_page(url) out_data = scraper.scrape(url, html=page['html']) if out_data['page classification'] == 'blog / essay': essays.append({ 'url': url, 'people thanked': out_data['people thanked'] })
Getting the Thanked People's Blogs
I then looped through all of the names and tried to search for a blog. I did a SERP request and then used an LLM to filter the correct results.
I packaged this up as webt.search_filter or POST /search/filter in the API.
import webtranspose as webt blog_dict = {} for person_name in people: results = webt.search_filter(f"{person_name}'s blog") if len(results['filtered_results']) > 0: blog_dict[person_name] = [x['url'] for x in results['filtered_results']]
Manual Linting
I then had to go through manually and lint the results.
There were a few people that didn't have great SEO, so I had to manually fix their job titles.
There were also some nicknames which I had to manually reconcile. Like "Yukihiro Matsumoto" is mentioned as "Matz" in the The Word "Hacker" essay.
I formatted the data as a Pandas DataFrame and then manually went through each row and linted the results.
Complete
You too can contribute to this dataset on Github here.
Or try scrape some websites yourself!